Prior-to-synthesis virtual discovery has long been inspired by the vastness of chemical spaces. In parallel research, promising candidate molecules or materials for further research are often sought out and classified based on a limited number of quantities deemed representative for the intended use. For first-principles computational screening approaches, it is common to measure such descriptors at predictive quality using electronic structure theory for each candidate in a chemical space or database that has been enumerated in some way. Initially conducted on small focused repositories, screening is now being applied to larger search spaces and, to more systematic and exhaustive enumerations within these spaces.
Unfortunately, even if based on computationally comparable undemanding descriptors, the combinatorial explosion characteristic of chemical versatility easily leads to unsolvable numbers of candidates for such comprehensive first-principles screenings. A computational funnel is a popular approach to this issue. Only the computationally least-demanding descriptors or even less demanding estimates of them are screened exhaustively in this case. Following that, the huge candidate set is restricted using the calculation of other descriptors and staged filtering only for smaller subsets that seem promising due to the previously calculated descriptors.
Unfortunately, chemical diversity indicates that the multi-objective (descriptor) landscape spanning the search space is rugged, with molecular or materials sub-classes possibly constituting different funnels and similar analogs leading to numerous local minima. This raises the question of whether such computational funneling will accurately identify the true optimum candidates.
Abandoning the original concept of exhaustively screening a previously identified chemical space or database is becoming a more appealing option. Instead, candidates resulting from an iteratively refining quest are limited to explicit first-principles computation of the descriptors. This is affordable in the context of data science by several learning principles, which also allow for the avoidance of predefining or a priori enumerating the search space. Few-shot learning, (semi-)supervised learning, and generative models are all examples.
Such concepts have already been applied successfully to drug discovery tasks to speed up molecular de novo design and drive autonomous discovery. Active machine learning (AML) has been researched as the most data-efficient approach for materials discovery based on first-principles descriptors.
AML utilizes acquired knowledge in the form of specifically calculated descriptors to successfully create a surrogate model of bigger and better regions of the rugged descriptor landscape. The predictive-quality calculations for recent candidates can then be balanced between exploration and exploitation in an iterative procedure.
In exploitation, the current surrogate model’s global experience is used to identify new promising candidates in a targeted manner. In exploration, Descriptors for new candidates are calculated explicitly to refine and expand the surrogate model. Gaussian Process Regression (GPR) and high values of its inherent Bayesian uncertainty calculation are utilized to identify candidates (or chemical space regions) where an explicit descriptor calculation would provide the latest information.
This concept is being followed in order to speed up the virtual exploration of organic semiconductors (OSCs) for electronic applications. OSCs are used in photovoltaics (OPVs), organic field effect transistors (OFETs), and light emitting diodes (OLEDs) and provide a wide range of properties as well as a low environmental and economic footprint. The spanned electronic property landscapes are considered to be extremely sensitive even to small molecular substitutions, and typical OSC-constituting molecules are of substantial size (e.g, 22 or 42 non-hydrogen atoms in the classic instances pentacene and rubrene, combined).
It is predicted that ~1033 similar-sized molecules can be synthesized, increasing the possibility that the currently recognized high-performing OSC molecular materials are just the tip of the iceberg. Several previous exhaustive screening or virtual discovery studies in somewhat restricted closed subspaces have been motivated by this.
A wide range of OSC molecules is examined in order to derive clear molecular-construction rules that encourage the generation of an in-principle limitless OSC chemical space. The AML discovery strategy then proceeds to explore this space, quickly identifying molecular candidates that outperform well-known OSC materials in terms of molecular electronic descriptors evaluating efficient charge injection and charge mobility.
Evaluating and analyzing the AML exploration within a chemical space network (CSN) comprising only a subsection of the design space, restricted to enable its complete enumeration, provides deep methodological insight. AML-discovery clearly outperforms a traditional funnel approach even within this truncated chemical space
The creation of a concise set of molecular construction rules that allows us to produce this space by iterative application is the foundation for our efficient AML exploration of a priori infinite molecular search space. Existing domain knowledge is used to examine the building blocks and motives found in molecules establishing several well-performing crystalline OSC molecular materials in order to create a diverse but problem-specific chemical space. For this analysis, the fact that most functionalized organic molecules can be easily disassembled into a molecular backbone (one or more cores), linkers (that bind cores), and side groups (attached to cores) is also utilized.
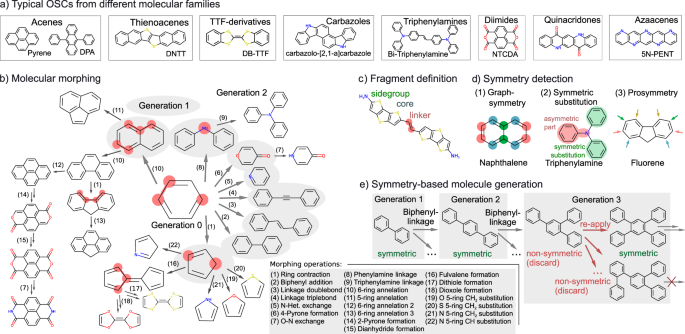
Reference:
- Kunkel, C., Margraf, J.T., Chen, K. et al. Active discovery of organic semiconductors. Nat Commun 12, 2422 (2021). https://doi.org/10.1038/s41467-021-22611-4
- https://pubmed.ncbi.nlm.nih.gov/33893287/
- https://www.newsbreak.com/news/2213860214569/active-discovery-of-organic-semiconductors