Summary: In present times we have seen machine learning deliver a number of incredible results but the failures it received cannot be left unseen. The failures encountered by and with machine learning have even ranged from totally harmless to potentially very deadly. Some new research also suggests that scientists and researchers may be mistaken in their assumption about why these failures and malfunctions happened. So more information and data is needed for a true evaluation of how reliable these networks of machine learning really are.
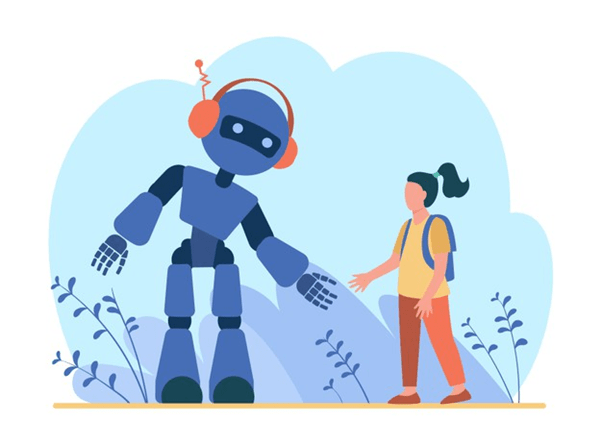
Full Story
Artificial intelligence has reached a cornerstone with deep and strong neural networks and the usage of mathematical modeling to process data and pictures through various multi-layered systems.
In present times we have seen machine learning deliver a number of incredible results but the failures it received cannot be left unseen. The failures encountered by and with machine learning have even ranged from totally harmless to potentially very deadly. Some new research also suggests that scientists and researchers may be mistaken in their assumption about why these failures and malfunctions happened. So more information and data are needed for a true evaluation of how reliable these networks of machine learning really are.
Artificial Intelligence in present times is capable of delivering results that seem sophisticated but they have also had many failures. The problem with artificial intelligence is that it can also be very easily fooled. This failure in reliability may be as harmless as their misidentification of one animal for another one, but it can be potentially deadly too. For example, if a self-driving car misidentifies a stop sign or a turn sign and still proceeds then the result can be deadly.
At UH an associate professor of Philosophy, Cameron Buckner explains how it is very important and the need of the hour for researchers to gain an understanding about the various sources of the failures that are so apparent and what they call adversarial examples. They call them adversarial examples when any data or image s misjudged by a neural network that is deep when the network gets to interact with something outside of what it saw during its training. They are called adversarial, and they are very rare because of the reason that often they get discovered or created by some other machine learning framework or system. He says this as his comment on how artificial intelligence and its many forms like machine learning are becoming a crucial part of our present world.
So we can say that the cause for a misfire in an AI could be how the actual patterns to be interpreted and the network that needs to interpret them interact with one another.
Cameron Buckner wrote in his paper and stated that in order to understand what the implications of adversarial examples are, an exploration of a third different possibility is essential. He then writes that in that way there is a risk in both just ignoring such patterns and continue the usage of it in a naive manner and it is a danger.
The highest risk is posed by intentional malfeasance and it majorly causes machine learning systems to get mistaken and lead to adversity. But it must be remembered that there are many other factors too that lead to the machine learning systems to make mistakes and misinterpret things. Buckner also adds, “It sums up that malicious actors could fool systems that rely on an otherwise reliable network. That has security applications.”
For example- there could be a breach of security when a security system that is based on facial recognition gets hacked. Someone can also place decals over various traffic signs on the road that may lead to a self-driving car to mistake the sign for something else. The biggest problem is that to a human eye the difference in the sign won’t even matter and be totally harmless.
It has also been found in several previous types of research that some natural adversarial situations may also occur like on times when a machine learning system makes a mistake because of a surprise interaction which was not anticipated by it. Such natural occurrences are rare but they do exist. This suggests that scientists and researchers need to pay more attention to why and how AI malfunctions and according to Buckner there is a “need to rethink how researchers approach the anomalies or artefacts.”
To understand these anomalies and artefacts Buckner gives a very simple analogy of how in a photograph, lens flares. This happens because of the interaction between the camera and the light and not due to any defect in the camera itself.
The lens flare can give us a number of useful information like the position of the sun. This according to Buckner raises the very important question on if the adversarial events that take place because of an anomaly could also have some useful and beneficial information that we can look into.
He also adds how it is also very important to understand that, “Some of these adversarial events could be artefacts.” He then adds how we need to know and understand all that we can about those artefacts to properly understand and be aware of such networks and their reliability.
Story Source:
Materials provided by the University of Houston.
Journal Reference:
Cameron Buckner. Understanding adversarial examples require a theory of artefacts for deep learning. Nature Machine Intelligence, 2020; DOI: 10.1038/s42256-020-00266-y